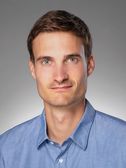
Dr. Malte Toetzke
Senior Research Fellow
Innovation and Entrepreneurship Research
Net Zero Lab
+49 89 24246-581
malte.toetzke(at)ip.mpg.de
Visit the Net Zero Lab:
Areas of Interest:
Natural Language Processing, Network Economics, Industrial Policy, Innovation and Entrepreneurship, Climate Tech, Climate Finance
Academic Résumé
Since 09/2024
Senior Research Fellow at the Max Planck Institute for Innovation and Competition (Innovation and Entrepreneurship Research), Net Zero Lab
Since 09/2024
Postdoc, TU Munich
05/2020 — 06/2024
Ph.D. Researcher, ETH Zurich
01/ 2023 — 03/2023
Visiting Ph.D. Researcher, University of Cambridge
09/ 2017 — 01/2020
M.Sc. Management, Technology and Economics, ETH Zurich
10/2012 – 08/2016
B.Sc. Industrial Engineering, Technical University of Dresden
09/2014 – 07/2015
Exchange year, University of Seville
Honors, Scholarships, Academic Prizes
2024 – 2026
TUM Global Postdoc Fellowship
2024 – 2025
Friedrich Schiedel Fellowship for Technology in Society
2024
FT Responsible Business Education Award, Financial Times
2023
HSG Impact Award
UNESCO Global Top AI Projects to Address the SDGs: Outstanding Project (Top 10)
2020
Google Research Grant
2015 – 2020
Merit Scholarship by the German Academic Scholarship Foundation (Studienstiftung des Deutschen Volkers)
2017 – 2019
Scholarship for foreign graduate studies by the German Academic Exchange Service (DAAD)
Publications
Articles in Refereed Journals
Systematic Assessment of the Achieved Emission Reductions of Carbon Crediting Projects, Nature Communications, 15. DOI
(2024).Leveraging Large Language Models to Monitor Climate Technology Innovation, Environmental Research Letters, 18 (9). DOI
(2023).- To achieve net-zero emissions, public policy needs to foster rapid innovation of climate technologies. However, there is a scarcity of comprehensive and up-to-date evidence to guide policymaking by monitoring climate innovation systems. This is notable, especially at the center of the innovation process, where nascent inventions transition into profitable and scalable market solutions. Here, we discuss the potential of large language models (LLMs) to monitor climate technology innovation. By analyzing large pools of unstructured text data sources, such as company reports and social media, LLMs can automate information retrieval processes and thereby improve existing monitoring in terms of cost-effectiveness, timeliness, and comprehensiveness. In this perspective, we show how LLMs can play a crucial role in informing innovation policy for the energy transition by highlighting promising use cases and prevailing challenges for research and policy.
Monitoring Global Development Aid with Machine Learning, Nature Sustainability, 5 (6), 533-541. DOI
(2022).- Monitoring global development aid provides important evidence for policymakers financing the Sustainable Development Goals (SDGs). To overcome the limitations of existing monitoring, we develop a machine learning framework that enables a comprehensive and granular categorization of development aid activities based on their textual descriptions. Specifically, we cluster the descriptions of ~3.2 million aid activities conducted between 2000 and 2019 totalling US$2.8 trillion. As a result, we generated 173 activity clusters representing the topics of underlying aid activities. Among them, 70 activity clusters cover topics that have not yet been analysed empirically (for example, greenhouse gas emissions reduction and maternal health care). On the basis of our activity clusters, global development aid can be monitored for new topics and at new levels of granularity, allowing the identification of unexplored spatio-temporal disparities. Our framework can be adopted by development finance and policy institutions to promote evidence-based decisions targeting the SDGs.
Consistent and Replicable Estimation of Bilateral Climate Finance, Nature Climate Change, 12 (19), 879-900. DOI
(2022).- International climate fnance is key to achieving the goals of the Paris
Agreement. Here we develop a machine learning classifer to identify
international climate fnance from 2.7 million ofcial development
assistance projects between 2000 and 2019, resulting in a consistent and
replicable inventory of 82,023 bilateral climate fnance projects (US$80
billion). Our fndings reinforce concerns that the actual numbers may be
much lower than current estimates made with Rio markers.
A Typology of Digital Building Technologies: Implications for Policy and Industry, IOP Conference Series: Earth and Environmental Science 323. DOI
(2019).- Digitalization and digital technologies are buzzwords in today's building industry. Because of their promising opportunities to improve (among others) the sustainability footprint of the built environment, they have emerged as an important topic for policymakers, managers, and researchers. Yet, the debate is dominated by references to Building Information Modelling (BIM) and to the success of digital businesses in other industries; it thereby fails to consider other promising digital building technologies and ignores that—in the building industry—many digital technologies require alignment with buildings' physical components. For these reasons, it is unclear how the implications of digital transformation of the building industry for policy and business. In this paper, we develop a typology of digital building technologies, and categorize and assess 29 important building technologies. The substantive differences among different types of building technologies provide valuable insights into how digital building technologies affect the functioning, structure, and competition in the building industry and where digital building technologies offer opportunities to remedy the industry's sustainability footprint. Based on our findings, we offer recommendations to policy makers, companies, and researchers interested in digital building technologies.
Conference papers
Mining Points-of-Interest Data to Predict Urban Inequality: Evidence from Germany and France, in: Ceren Budak, Meeyoung Cha, Daniele Quercia (
- Reducing inequality is a major goal of the Sustainable Development Goals. Inequality is many-sided and often appears across geographic boundaries. Urban inequality refers to inequality between urban neighborhoods. Despite close distances, it reveals considerable disparities in income level, unemployment rates, and other socio-economic indicators and is highly dangerous for democratic societies. However, little is known about determinants indicating urban inequality. Here, we propose to explain urban inequality based on point-of-interest (POI) data from the online platform Open Street Maps. For this, we leverage machine learning to predict three major indicators of urban inequality, namely, unemployment rate, income level, and foreign national rate. We evaluate our machine learning approach using POI data for neighborhoods in Paris, Lyon, Marseille, Berlin, Hamburg, and Bremen. We find: (1) POIs are highly predictive of intra-city inequality explaining up to 75% of out-of-sample variance of urban inequality. (2) POIs generalize across cities and, thereby, can help to explain urban inequality in other cities, where no socio-economic data is available. (3) Important POIs for the prediction model are, e.g., banks and playgrounds. To the best of our knowledge, our work is the first to show urban inequality through POIs. As such, POIs can be used to infer granular mappings of urban inequality and thereby provide cost-effective evidence for policy-makers.
- https://doi.org/10.1609/icwsm.v16i1.19286
- Event: International AAAI Conference on Web and Social Media, Atlanta, Georgia, USA, 2022-06-06
Contributions to Collected Editions
Chapter 2: Research and Development, in: S.M. Smith (
Further Publications, Press Articles, Interviews
Mit KI den nachhaltigen Wandel gestalten - Zur strategischen Verknüpfung von Künstlicher Intelligenz und Nachhaltigkeitszielen. München: acatech, Deutsche Akademie der Technikwissenschaften. DOI
(2022).Discussion Papers
Can Deep Learning Help to Forecast Deforestation in the Amazonian Rainforest? - Paper presented at the NeurIPS 2023 Workshop: Tackling Climate Change with Machine Learning.
(2023).- Deforestation is a major driver of climate change. To mitigate deforestation, carbon offset projects aim to protect forest areas at risk. However, existing literature shows that most projects have substantially overestimated the risk of deforestation, thereby issuing carbon credits without equivalent emissions reductions. In this study, we examine if the spread of deforestation can be predicted ex-ante using Deep Learning (DL) models. Our input data includes past deforestation development, slope information, land use, and other terrain- and soil-specific covariates. Testing predictions 1-year ahead, we find that our models only achieve low levels of predictability. For pixel-wise classification at a 30 m resolution, our models achieve an F1 score of 0.263. Only when substantially simplifying the task to predicting if any level of deforestation occurs within a 1.5 km squared tile, the model results improve to a moderate performance (F1: 0.608). We conclude that, based on our input data, deforestation cannot be predicted accurately enough to justify the ex-ante issuance of carbon credits for forest conservation projects. As main challenges, there is the extreme class imbalance between pixels that are deforested (minority) and not deforested (majority) as well as the omittance of social, political, and economic drivers of deforestation.
- https://www.climatechange.ai/papers/neurips2023/22
Invited Talks
06.12.2023
Potential of Natural Language Processing Tools to Monitor Technology Innovation
Expert Presentation, 62nd Meeting of the Working Party on Innovation and Technology Policy, OECD
Location: Paris, France
16.11.2023
Monitoring Innovation Networks around Climate Technologies from Social Media Announcements via Large Language Models
Research Seminar, Mercator Institute on Global Commons and Climate Change (MCC)
Location: Berlin
28.02.2023
Checking Contentious Counting: How Machine Learning Can Help to Verify International Climate Finance
AI4ER Seminar, University of Cambridge
Location: Cambridge, UK
26.01.2023
Using Natural Language Processing to Inform Public Policy in the Energy Transition
CEENRG Seminar, University of Cambridge
Location: Cambridge, UK
31.05.2022
Supervised and Unsupervised Machine Learning Methods to Inform Development Policies
ETH NADEL Advanced Seminar for Leaders in Public Organizations
Location: Zurich, Switzerland
19.05.2022
Analyzing the Newspaper Discourse Around Clean Energy Technologies
International Research Workshop on AI in Climate Politics, hosted at ETH Zurich
Location: Zurich, Switzerland
02.05.2022
Machine Learning in Global Development
Presentation to the German AI Delegation organized by the Swiss Foreign Office
Location: Zurich, Switzerland
03.02.2022
Monitoring Global Development Aid with Machine Learning
AI-Keynotes Seminar Series, LMU Munich and University of Cologne
Location: online